备注
单击 here 下载完整的示例代码或通过活页夹在浏览器中运行此示例
边运算符¶
在边缘检测算法中,边缘算子被用于图像处理。它们是离散微分运算符,计算图像强度函数的梯度的近似值。
import numpy as np
import matplotlib.pyplot as plt
from skimage import filters
from skimage.data import camera
from skimage.util import compare_images
image = camera()
edge_roberts = filters.roberts(image)
edge_sobel = filters.sobel(image)
fig, axes = plt.subplots(ncols=2, sharex=True, sharey=True,
figsize=(8, 4))
axes[0].imshow(edge_roberts, cmap=plt.cm.gray)
axes[0].set_title('Roberts Edge Detection')
axes[1].imshow(edge_sobel, cmap=plt.cm.gray)
axes[1].set_title('Sobel Edge Detection')
for ax in axes:
ax.axis('off')
plt.tight_layout()
plt.show()
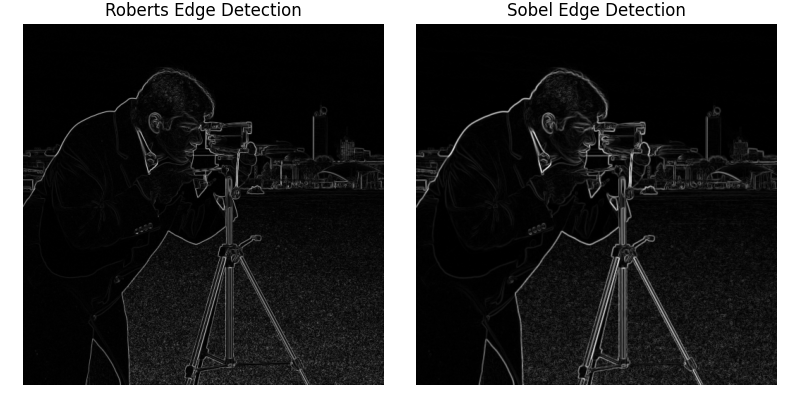
不同的运算符计算不同的梯度有限差分近似。例如,Scharr滤波器产生的旋转变化小于Sobel滤波器,而Sobel滤波器又好于Prewitt滤波器 1 2 3. Prewitt和Sobel过滤器与Scharr过滤器之间的区别如下所示,该图像是旋转不变连续函数的离散化。对于图像中梯度方向接近对角线的区域,以及具有高空间频率的区域,Prewitt和Sobel过滤器与Scharr过滤器之间的差异更大。对于示例图像,过滤结果之间的差异非常小,并且过滤结果在视觉上几乎无法区分。
- 1
Https://en.wikipedia.org/wiki/Sobel_operator#Alternative_operators
- 2
B.Jaehne,H.Scharr和S.Koerkel.滤光片设计原则。《计算机视觉与应用手册》。学术出版社,1999。
- 3
x, y = np.ogrid[:100, :100]
# Creating a rotation-invariant image with different spatial frequencies.
image_rot = np.exp(1j * np.hypot(x, y) ** 1.3 / 20.).real
edge_sobel = filters.sobel(image_rot)
edge_scharr = filters.scharr(image_rot)
edge_prewitt = filters.prewitt(image_rot)
diff_scharr_prewitt = compare_images(edge_scharr, edge_prewitt)
diff_scharr_sobel = compare_images(edge_scharr, edge_sobel)
max_diff = np.max(np.maximum(diff_scharr_prewitt, diff_scharr_sobel))
fig, axes = plt.subplots(nrows=2, ncols=2, sharex=True, sharey=True,
figsize=(8, 8))
axes = axes.ravel()
axes[0].imshow(image_rot, cmap=plt.cm.gray)
axes[0].set_title('Original image')
axes[1].imshow(edge_scharr, cmap=plt.cm.gray)
axes[1].set_title('Scharr Edge Detection')
axes[2].imshow(diff_scharr_prewitt, cmap=plt.cm.gray, vmax=max_diff)
axes[2].set_title('Scharr - Prewitt')
axes[3].imshow(diff_scharr_sobel, cmap=plt.cm.gray, vmax=max_diff)
axes[3].set_title('Scharr - Sobel')
for ax in axes:
ax.axis('off')
plt.tight_layout()
plt.show()
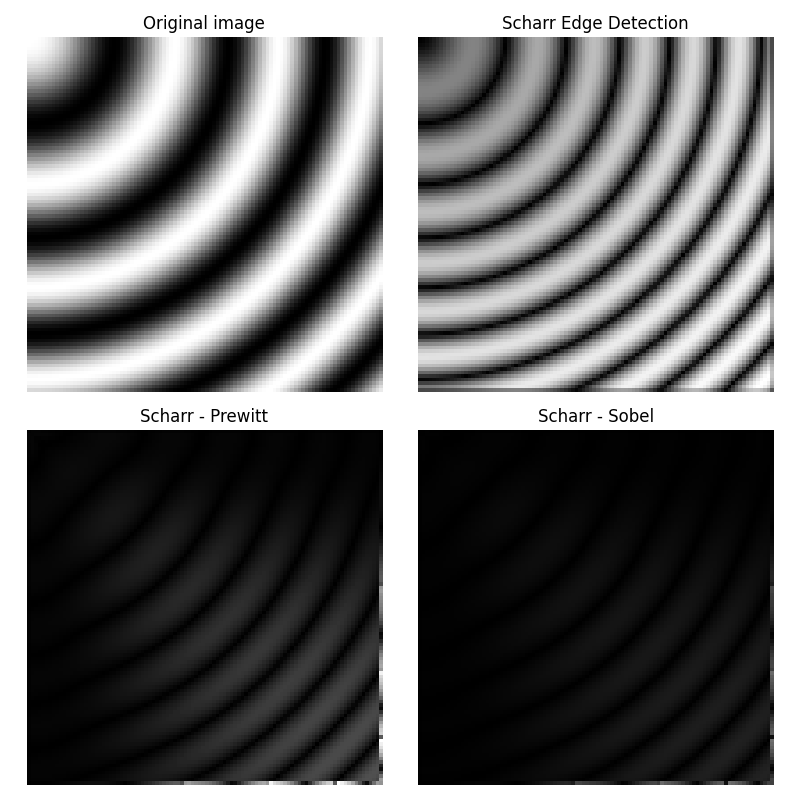
与前面的例子一样,我们在这里说明滤光片的旋转不变性。顶行显示了一幅旋转不变的图像及其解析梯度的角度。另外两行包含不同的梯度近似(Sobel、Prewitt、Scharr&Farid)和解析梯度之间的差异。
Farid&Simoncelli导数滤波 4, 5 是最具旋转不变性的,但需要5x5内核,这在计算上比3x3内核更密集。
- 4
法里德,H.和西蒙塞利,E.P.,“离散多维信号的微分”,IEEE图像处理学报13(4):496-508,2004。 DOI:10.1109/TIP.2004.823819
- 5
维基百科,“法里德和西蒙塞利的衍生品。”可在以下网址获得:<https://en.wikipedia.org/wiki/Image_derivatives#Farid_and_Simoncelli_Derivatives>
x, y = np.mgrid[-10:10:255j, -10:10:255j]
image_rotinv = np.sin(x ** 2 + y ** 2)
image_x = 2 * x * np.cos(x ** 2 + y ** 2)
image_y = 2 * y * np.cos(x ** 2 + y ** 2)
def angle(dx, dy):
"""Calculate the angles between horizontal and vertical operators."""
return np.mod(np.arctan2(dy, dx), np.pi)
true_angle = angle(image_x, image_y)
angle_farid = angle(filters.farid_h(image_rotinv),
filters.farid_v(image_rotinv))
angle_sobel = angle(filters.sobel_h(image_rotinv),
filters.sobel_v(image_rotinv))
angle_scharr = angle(filters.scharr_h(image_rotinv),
filters.scharr_v(image_rotinv))
angle_prewitt = angle(filters.prewitt_h(image_rotinv),
filters.prewitt_v(image_rotinv))
def diff_angle(angle_1, angle_2):
"""Calculate the differences between two angles."""
return np.minimum(np.pi - np.abs(angle_1 - angle_2),
np.abs(angle_1 - angle_2))
diff_farid = diff_angle(true_angle, angle_farid)
diff_sobel = diff_angle(true_angle, angle_sobel)
diff_scharr = diff_angle(true_angle, angle_scharr)
diff_prewitt = diff_angle(true_angle, angle_prewitt)
fig, axes = plt.subplots(nrows=3, ncols=2, sharex=True, sharey=True,
figsize=(8, 8))
axes = axes.ravel()
axes[0].imshow(image_rotinv, cmap=plt.cm.gray)
axes[0].set_title('Original image')
axes[1].imshow(true_angle, cmap=plt.cm.hsv)
axes[1].set_title('Analytical gradient angle')
axes[2].imshow(diff_sobel, cmap=plt.cm.inferno, vmin=0, vmax=0.02)
axes[2].set_title('Sobel error')
axes[3].imshow(diff_prewitt, cmap=plt.cm.inferno, vmin=0, vmax=0.02)
axes[3].set_title('Prewitt error')
axes[4].imshow(diff_scharr, cmap=plt.cm.inferno, vmin=0, vmax=0.02)
axes[4].set_title('Scharr error')
color_ax = axes[5].imshow(diff_farid, cmap=plt.cm.inferno, vmin=0, vmax=0.02)
axes[5].set_title('Farid error')
fig.subplots_adjust(right=0.8)
colorbar_ax = fig.add_axes([0.90, 0.10, 0.02, 0.50])
fig.colorbar(color_ax, cax=colorbar_ax, ticks=[0, 0.01, 0.02])
for ax in axes:
ax.axis('off')
plt.show()
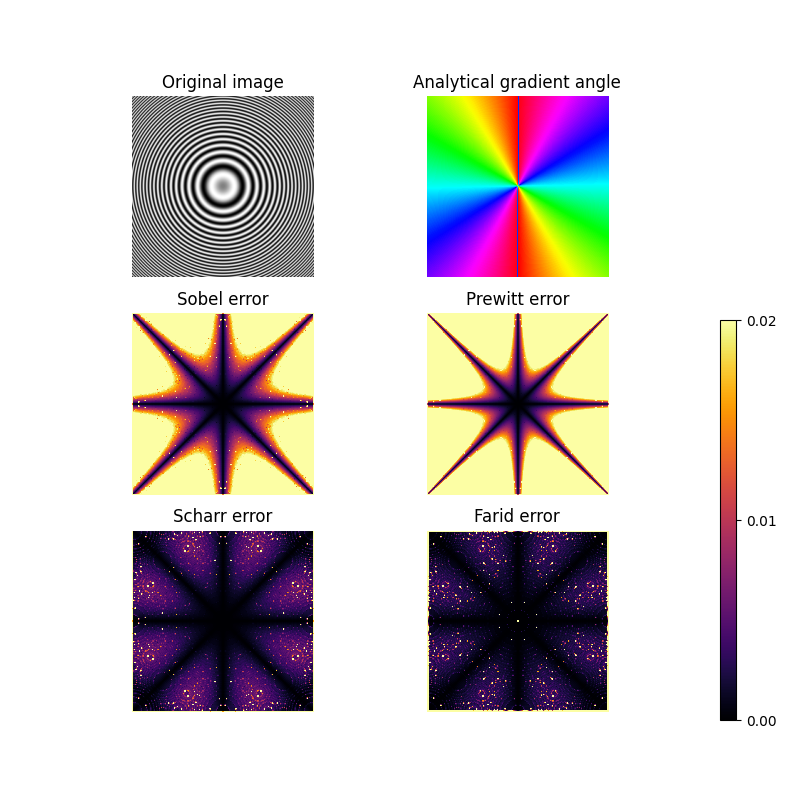
脚本的总运行时间: (0分0.522秒)